Overview
Data is the new gold, and skills related to data are highly sought after. In its most common form, Artificial Intelligence and Machine Learning combine a set of techniques that help us to make predictions based on a set of ‘example’ outcomes or labels, given specific inputs. Learning from labelled data examples is called supervised learning. In many cases, however, one cannot rely on labelled data, simply because it is not available. These types of problems are called unsupervised learning problems. Both supervised and unsupervised learning are very relevant and are applicable in almost every industry. One way to deal with unsupervised data is to label the data and convert it to a supervised learning problem. Data labelling, however, can be time-consuming, complex and expensive. In this course, the focus is on native unsupervised learning applications such as Anomaly Detection and Clustering. Clustering groups data with similar characteristics while in Anomaly Detection, the aim is to find data that is considered out of range. Both unsupervised learning applications are very relevant and are used by almost all verticals. Manufacturing companies rely on Anomaly Detection to detect quality issues while banks use it to detect fraudulent transactions.
Recommender Systems used by Amazon, and Netflix are examples of what we call semi-supervised learning applications, because they rely on a small percentage of labelled data. Reinforcement Learning (RL) or learning by doing is also unsupervised and, initially, there are no examples to learn from. The RL agent generates its own examples, over time and adds to the complexity of RL problems. Finally, the course will cover Time Series problems. These types of problems rely on supervised but sequential data.
Course Description & Learning Outcomes
At the end of this course, learners will be able to:
• Gain an understanding of popular unsupervised and semi-supervised learning problems including Clustering, Anomaly Detection and Recommender Engines.
• Recognise unsupervised learning problems
• Solve real business problems, including customer segmentation, quality control and fraud detection use cases, using RapidMiner.
• Understand bias and that some supervised classification problems are better solved via Anomaly Detection.
• Make a dataset ‘Artificial Intelligence-ready’ using RapidMiner.
• Solve real business problems associated with time series or sequential data using RapidMiner.
• Understand the complexities of reinforcement learning and dealing with dynamic systems.
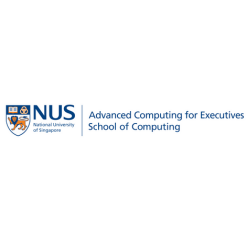
Schedule
End Date: 07 Jun 2024, Friday
Location: 11 Research Link COM 3, 119391
Agenda
Day/Time | Agenda Activity/Description |
---|---|
5 Jun, 6 Jun, 7 Jun 2024 | 9am – 5.30pm | Business Applications Relying on Unsupervised & Reinforcement Learning |
Pricing
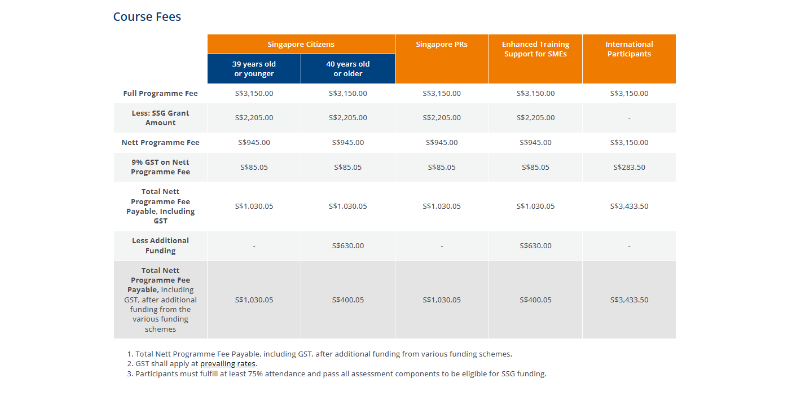