Why You Need Machine Learning Operations to Achieve AI Governance
Mon, 07/27/2020 - 12:00
Discussions on trust in Artificial Intelligence (AI) often do not lead to tangible action. Ethics principles are supposed to help define the boundaries and responsibilities for action, especially in highly ambiguous situations. Often, they remain amorphous, doing exactly the opposite of its intent.
Yet the use of AI will invariably bring about ethical dilemmas. And trust is at the heart of corporate reputation. From the boundaries of privacy to the impact of social media algorithms on our mental and emotional wellbeing, ethical decisions need to be made by leaders, not machines. This entails a moral judgement on what is good, and what is harmful. For example, how do we define a fair decision-making process? What features (e.g. gender, age, income) are justifiable in the delivery of differential treatment or services?
How can enterprises pursue AI and uphold trust?
“A strong AI governance framework is how AI adoption can be accelerated and used responsibly. It is the foundation for sustainable AI growth.”
At the heart of trust in AI, is a sound AI governance framework that is based on clear ethical principles. It is typically articulated as a set of principles that can be translated into actions, processes, and metrics to guide the use of AI in a way that is explainable, transparent, and ethical. When integrated with other parts of the organisation, decision trade-offs can be made in view of overall compliance and risk management perspectives.
Is this even possible? The devil is in the details.
In reality, there is a gulf between the governance concepts that policymakers describe, and the technical processes required to build these AI systems. AI systems struggle to make it to production stage because things are lost in translation when the model is “thrown over the wall” from data scientists to the Machine Learning engineer. The use of black-box models can be a double-edged sword—they are quick to plug and play, but enterprises are blind to what the algorithm is doing or what methods it is using to generate the prediction.
MLOps (a compound of “Machine Learning” and “operations”) has proved a useful foundation for the implementation of sound AI governance. The approach arose out of the recognition that it is challenging to build a performant Machine Learning engine and subsequently maintain them. It is an emergent Machine Learning practice that draws from DevOps approaches to increase visibility, automation, and transparency in Machine Learning systems.
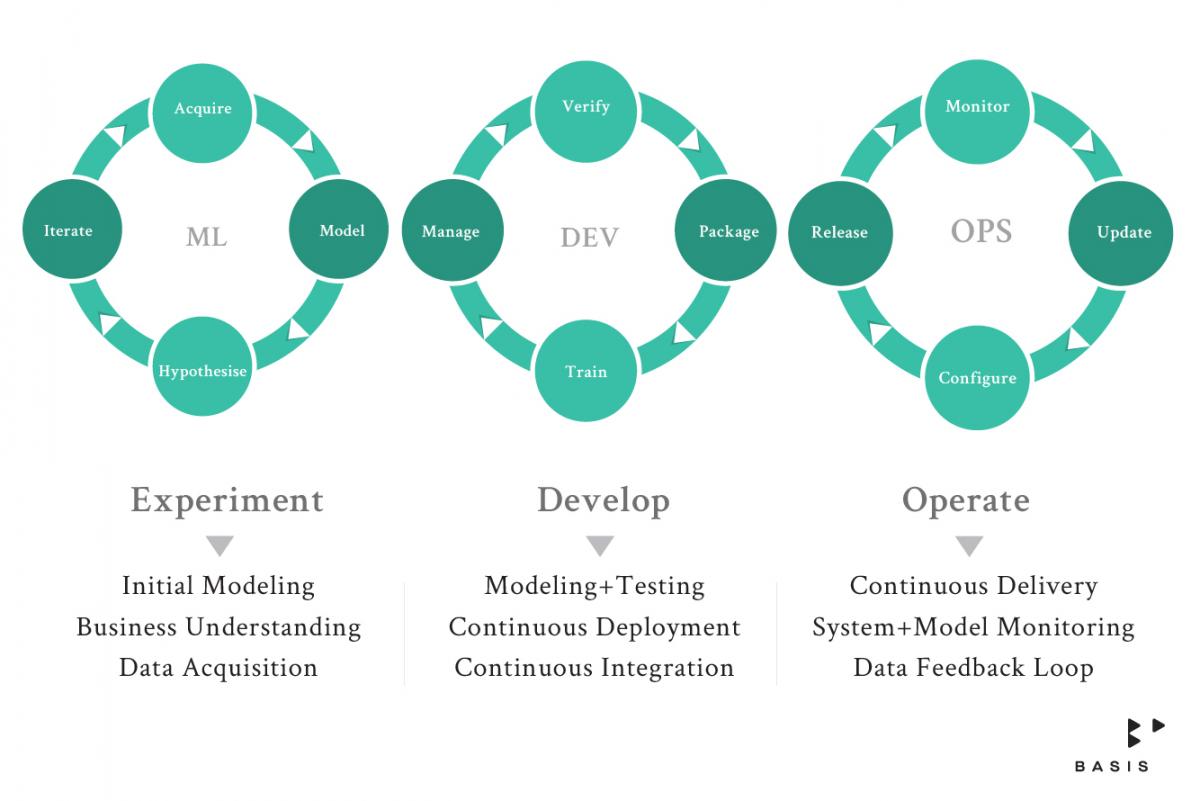
MLOps strengthens the transparency of AI systems through visibility of metrics. By enabling users to monitor and understand the way the AI systems are functioning at every level, ethical trade-offs become more transparent. For example, we can compare a model designed to have maximum effectiveness but no fairness constraints, versus another model that is designed to be fair, to see the relative drop ineffectiveness.
It also provides a systematic way to detect bias and avoid ethical mishaps. AI systems are built on data that is evolving as the environment changes. Ongoing monitoring is necessary to ensure that the system is still working as intended, and biases have not quietly crept in. With version control, MLOps provides users to monitor for these potential kinks, and course-correct or roll back where necessary. By automating tasks in the model development and deployment process, MLOps also enhances the productivity of data scientists and engineers so that they can focus on the creative aspects.
Even though MLOps for effective model management has only emerged in the last couple of years, it has been predicted to be a major trend in the next few years, where the ML Ops market will be over $4 Billion, making it a significant component of the AI solution landscape.
The concerns over trust in the use of AI cannot be addressed solely by technology. It must be accompanied by AI governance, which is an intersection of two practices: one originating from ethics; the other from engineering processes such as MLOps. Proactive governance and accountability practices are increasingly seen as a differentiating factor for businesses to brand themselves as trustworthy. Investing early in a sound AI governance framework and a robust MLOps process will serve as a solid foundation for enterprises to accelerate AI adoption.
At SGInnovate, we believe that AI and Machine Learning will be the bedrock of future technologies. This is why ethics and governance are conversations we always have at our events.
Download Basis AI’s white paper here to read more on AI governance.
Trending Posts
- The simple blood draw that tests for 50 cancers long before symptoms develop
- Taking the first steps towards advancing precision health in Asia
- How his biotech internship is delivering impact and unlocking industry insights
- Singapore’s Deep Tech ecosystem at a glance
- Start smart: Leader in molecular cell biology and biotech research Harvey Lodish shares tips for entrepreneurs