When AI Gets Leaner and Meaner
Thu, 02/22/2018 - 12:00
New AI approaches that require less data and computational power are changing the game for tech giants and startups alike, said speakers at the INSEAD Artificial Intelligence and Machine Learning Festival.
In the early 1990s—before the internet went mainstream—Professor John Little of the Massachusetts Institute of Technology was already thinking about how managers could use data to make timely marketing decisions. Widely regarded as the father of marketing science, he developed algorithms to automatically analyse scanner data—sales information obtained by scanning product barcodes at the cash register—and provide managers with informed insights.
This problem-driven approach—a departure from early computer programmes, which mostly used classical statistics to analyse data—is also what underpins the artificial intelligence (AI) and machine learning strategies in use today, said Professor Phil Parker, chaired professor of management science at INSEAD.
"Today, if you don’t start with a very concrete objective, you may find yourself in a situation where you invest a lot of money in big data, and two years later, you wonder how to monetise it,” said Professor Parker.
The best way is to start with a problem and then reverse engineer the proper algorithms.
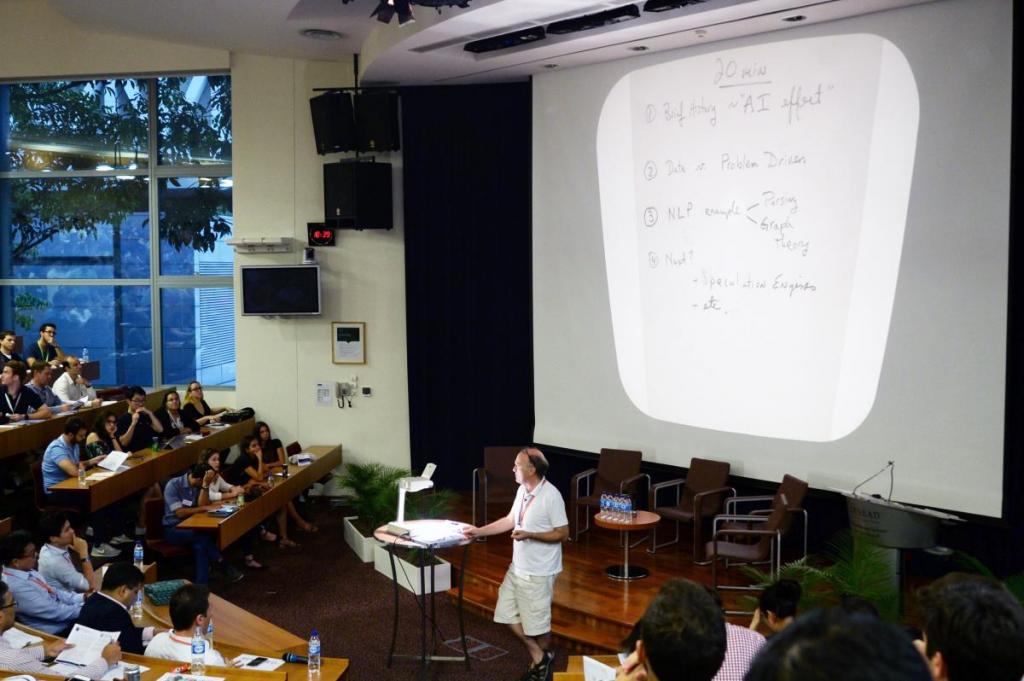
Professor Parker stressed the need for a concrete objective as a starting point
Professor Parker was speaking on 4 December 2017 at the Artificial Intelligence and Machine Learning Festival, a three-day event organised by INSEAD, SGInnovate and Impact Hub. He was joined that evening by domain experts from industry and government, who spoke on recent developments in the field of AI and their wide-ranging implications for society, businesses and the economy.
Putting AI advances into practice
In addition to becoming more problem-driven, AI has also advanced in other areas, said Mr Samuele Saini, head of strategy and insights at Google Singapore. For example, while traditional machine learning methods require large, labelled datasets on which to train, researchers are now making headway in teaching computers to learn from scratch.
Large datasets are certainly a great help, but they are not a requirement.
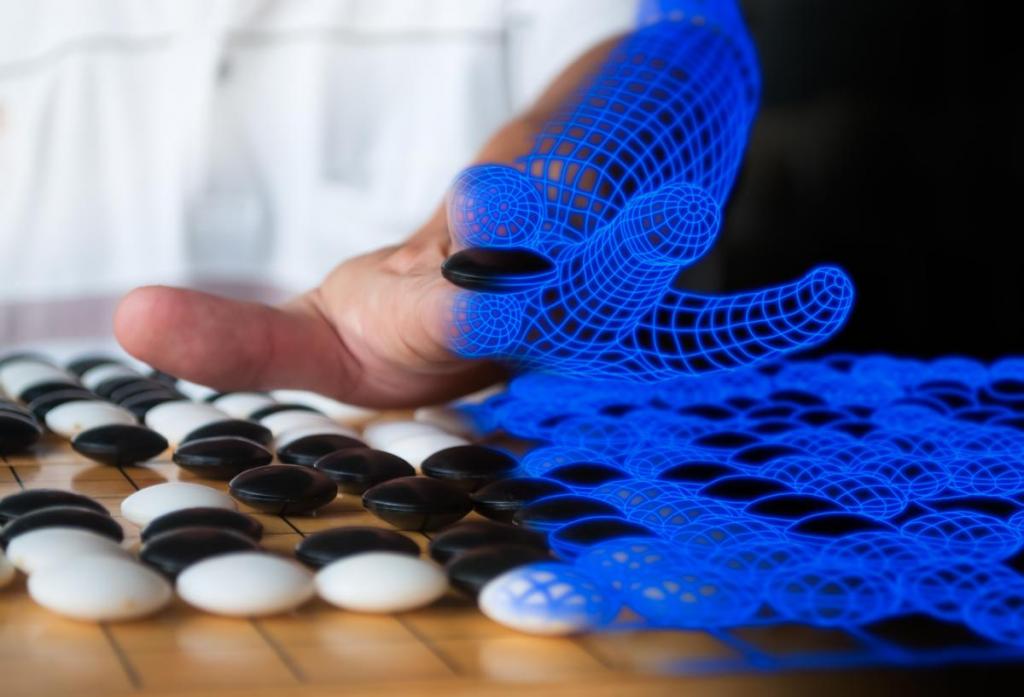
DeepMind’s AlphaGo Zero, for instance, used reinforcement learning to train itself to play Go without any human input; within 40 days, the programme was playing at world-champion level. Besides relying less on large datasets, AI approaches now also make use of machine learning techniques that require less computational power, added Mr Saini.
Google has applied these advances to numerous consumer-facing products and other aspects of its business, including search, image recognition, recommended email replies and providing insights to advertisers, continued Mr Saini. Google’s translation service, in particular, saw a dramatic improvement almost overnight when the company decided to experiment with neural networks instead of the incumbent method of statistical machine translation.
“In one month, the system improved by the same order of magnitude as it had over the previous five years. Google decided to stop development of the previous [statistical machine translation] system and move completely into neural machine translation,” he explained. The system can now translate sentences as a whole instead of doing so word by word, as well as translate directly from one language to another without having to go through an English intermediate, he added.
With its countless applications, AI has been a game-changer for Google and other tech giants.
“We moved from being a mobile-first company to being an AI-first company—that’s really what is giving us our competitive advantage going forward", said Mr Saini.
The rise of the startups
While large companies now dominate the AI scene, the innovation going on in startups should not be overlooked, said Mr Sushil Anand, cognitive lead for ASEAN at IBM. Although tech giants are now indispensable by virtue of the fact that they provide the platforms—operating systems and cloud computing, for example—on which other businesses operate, smaller companies can still carve out their own niches by developing novel applications, he explained.
“For example, although the Android operating system was launched by Google, mobile apps were able to make money off it,” he said.
Startups are key for solving business-specific problems.
Indeed, technology research firm Gartner predicts that by 2019, startups will overtake the likes of Amazon, Google, IBM and Microsoft in “driving the AI economy with disruptive business solutions”. Further, added Mr Anand, venture capitalists are investing most heavily in industry-specific AI applications—trade financing, health care and claims processing, for instance—areas where startups can shine.
Despite their promise, startups face difficulties of their own—most are unable to match the high salaries offered by tech giants, and thus find it a challenge to hire AI talent, said Mr Laurence Liew, director of AI industry innovation at the government-led AI Singapore initiative.
AI Singapore has thus implemented measures to give smaller companies a leg up. The 100 Experiments (100E) programme, for example, will match companies with AI researchers in institutes of higher learning, according to the AI problem they are trying to solve, said Mr Liew.
The democratisation of AI
Revisiting the idea of machines being able to train on little or no data, Mr Liew commented that this approach more closely mimics the way humans learn. “Today, if you want AI to recognise a cat, you need 10,000 images of a cat. But if you think about it, how many cats do you need to show your child before he or she learns that it’s a cat and not a dog? Certainly not 10,000—three or four would be enough.”
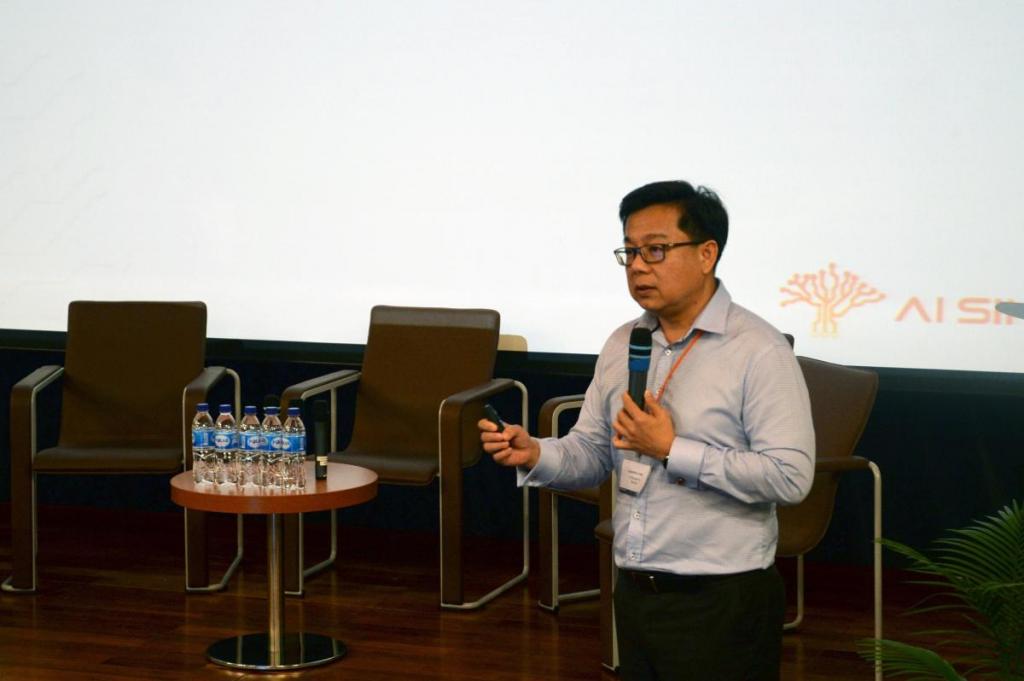
Mr Liew speaking to the crowd at the INSEAD campus about the democratisation of AI
As more breakthroughs are made in this area, giant companies that have accumulated enormous repositories of user data will no longer hold such an outsized advantage in the AI space, said Mr Liew.
“AI will become even more pervasive—any company with the right technology will be able to perform it because there will no longer be a need to collect tens of thousands of images,” he predicted. “Just imagine the power of a system that can perform image recognition with just a couple of objects; the same goes for text or for any sort of prediction.”
Artificial Intelligence (AI) is one of SGInnovate’s focus areas under the Deep Tech Nexus strategy. Check out our AI events and more here and stay connected with us via our Facebook, Twitter & LinkedIn.
Trending Posts
- How this quantum cybersecurity startup is enabling businesses to stay ahead of the curve
- Mapping Singapore’s early-stage emerging technology startups
- How GDMC’s Brian Liau is fostering tomorrow’s gene therapy talent through a biotech mentorship programme
- The uphill journey of building a startup: Fabrica AI’s founders share key lessons
- Steps to build an impactful career in science according to these Nobel Prize winners